[ad_1]
Artificial intelligence (AI) is already transforming the global economy. Companies are investing hundreds of billions of dollars each year in these technologies. In almost every sector, AI is being used to drive operational efficiencies, manage complexity, provide personalized services and speed up innovation.
As AI’s influence on society grows, questions arise about its impact on greenhouse-gas emissions: will its myriad applications help to reduce the world’s carbon footprint or hinder climate progress? The answer will depend on how AI models are developed and operated, and what changes result from their use. And scientists simply don’t know how all that will pan out — a worrying situation when there is so much at stake.
Most discussions so far about AI’s environmental consequences have focused on the direct impacts of these computationally intensive technologies — how much energy, water or other resources they consume and the amount of greenhouse gases they generate. But the global repercussions of AI applications for society will be much broader, from transforming health care and education to increasing the efficiency of mining, transportation and agriculture.
Such AI-driven changes can lead to indirect effects on emissions, which might be positive or negative. These indirect effects also need to be taken into account, and could vastly exceed those from direct impacts1,2. Assessments of all types of AI impact are urgently needed. Here’s what we know and what we don’t.
Uncertainty ahead
The direct impacts of AI on climate so far are relatively small. AI operations for large models require millions of specialized processors in dedicated data centres with powerful cooling systems. AI processors installed in 2023 consume 7–11 terawatt hours (TWh) of electricity annually, which is about 0.04% of global electricity use3. That is less than for cryptocurrency mining (100–150 TWh) and conventional data centres (500–700 TWh), which together accounted for 2.4–3.3% of global electricity demand in 2022, according to the International Energy Agency (IEA). Thus, in terms of total global greenhouse-gas emissions, we calculate that AI today is responsible for about 0.01%, on the basis of IEA assessments showing that data centres and transmission networks together account for about 0.6% (see go.nature.com/3q7e6pv).
AI use is expanding rapidly. Over the past decade, the compute capacity used to train advanced large language models has increased tenfold each year. Demand for AI services is expected to rise by 30–40% annually over the next 5–10 years. And more powerful AI models will require more energy. One estimate suggests that, by 2027, global AI-related energy consumption could be 10 times greater than it was in 20233, or about as much as is consumed annually by people watching television in US homes. Although there could be challenges for local electricity grids in regions where many data centres are based, from a global perspective, AI should not lead directly to large, near-term increases in greenhouse-gas emissions.
Improvements in energy efficiency could offset some of the projected increase in power demand, as they did when data centres expanded in the 2010s4. More-efficient AI algorithms, smaller models and innovations in hardware and cooling systems should help5,6. For example, small language models, such as Microsoft’s Phi-2 and Google’s Gemini Nano, can run on mobile phones and deliver capabilities previously seen only with the largest models. AI companies are increasingly investing in renewable power and setting up operations in countries or regions with abundant clean-energy supplies, such as Iceland.
Indirect effects are less clear, however. Some AI applications are designed to tackle climate change, for example to reduce emissions from the energy and transport sectors, from buildings and industry operations and from land use. Optimizing supply chains will make manufacturing more efficient and support the integration of renewable energy into electricity grids. Speeding up the development of new materials for batteries and renewable energy7,8 will be a boon.
There could also be some negative indirect impacts. Embedding AI into existing applications, from health care to entertainment, might drive more electricity use. Oil and gas exploration and extraction could become cheaper, potentially driving up production. And without proper governance, the widespread use of AI could affect political and economic stability, with ramifications for poverty, food security and social inequalities — all of which could have knock-on effects for emissions9.
And that’s just existing AI systems. How will future AI technologies develop? How will their expansion affect the global economy? And how will this affect decarbonization? Researchers simply don’t know; it’s too early to tell. It is tempting to simply extrapolate past AI electricity-use trends into the future, but overlooking social, economic and technological factors often results in large forecasting errors4,5,10. Similarly, an overly simplistic view of the impacts of indirect emissions risks underestimating AI’s potential for accelerating important climate-solution breakthroughs, such as the development of less expensive and more powerful batteries in months rather than decades11.
AI-driven emissions scenarios
Recognizing these huge uncertainties, here we call on researchers to develop a set of policy-relevant scenarios to quantify the effects that AI expansion could have on the climate under a range of assumptions. Routinely used by financial institutions to understand risks and opportunities and plan investments, scenarios combine quantitative models with expert consultations. Rather than making predictions, they explore many possible futures based on influential factors.
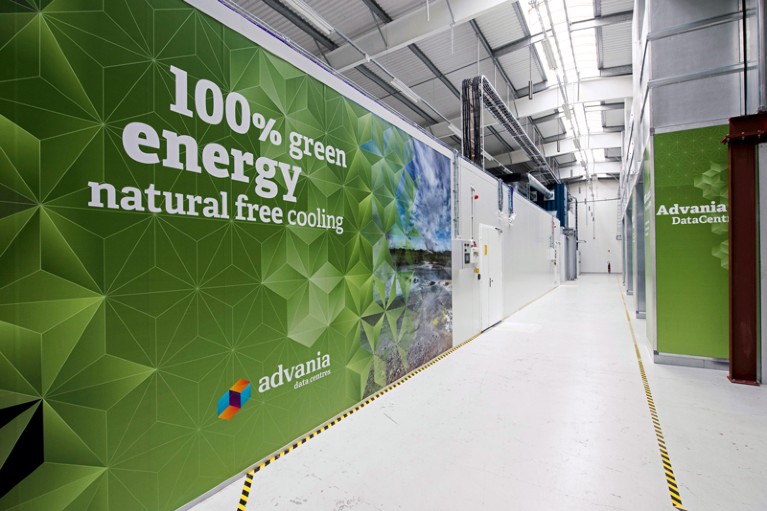
A data centre near Reykjavik uses renewable energy for cooling.Credit: Sigtryggur Ari/Reuters
Specifically, we recommend that a suite of scenarios be built to better understand how AI expansion might affect emissions, both directly and indirectly. These scenarios should range from a ‘reference’ case without widespread adoption of powerful AI technologies, to an ‘aspirational’ case in which all the United Nations Sustainable Development Goals are achieved; scenarios should also include ones with undesirable outcomes.
Five elements are essential for AI-driven emissions scenarios to be credible and useful.
Link to existing climate scenarios. The climate community already uses integrated assessment models (IAMs) to assess future greenhouse-gas emissions quantitatively on the basis of qualitative narratives about potential socio-economic, demographic, policy, technology and governance outcomes. Five standard scenarios, or Shared Socioeconomic Pathways (SSPs), are widely used. These range from a future in which the world is deeply divided and remains hooked on fossil fuels to a more optimistic scenario of global cooperation, decoupling of economic growth from emissions and serious investment in clean energy.
AI should be integrated into these pathways, along with the global shocks and technological breakthroughs that might accompany it. This would require major work, including incorporating expertise from the AI community, rethinking each of the pathway narratives and exploring whether new ones need to be added. Could AI take the world to a more radically green future, or a more dystopian one? What factors define those outcomes? How plausible are they? Scenarios can help to narrow down answers.
Turning these narratives into quantitative scenarios will require developing new analytical models, collecting new types of data and establishing an institutional structure to enable rapid updates to keep up with the fast pace of societal transformations that AI is driving, as we outline here.
Develop quantitative analytical frameworks. Developing IAMs for exploring the influence of AI will require improved data and analytical frameworks for both direct and indirect impacts. The biggest challenge will be quantifying the range of indirect effects resulting from AI-driven societal transformations, as well as AI-powered innovations on climate-relevant advances and breakthroughs.
For example, AI personalization could encourage sustainable consumption, but it could also increase demand for resource-intensive goods. And disentangling the emissions impacts of AI-enabled innovations from other technologies that lower emissions, such as renewables or carbon capture, will be challenging because the pace of research and development differs across sectors. Policies and regulations are also often slow to catch up. Quantifying the interplay of these dynamics will be difficult.
Comparing and replicating scenarios will be key to improving them as AI systems are rolled out. Researchers should regularly run comparisons between different models for direct and indirect AI-related emissions, coordinated through platforms used by the climate community, such as the Energy Modeling Forum and the Integrated Assessment Modeling Consortium. Scientists must ensure that the data and assumptions in these analyses are fully documented, freely shared and completely replicable by others.
Share data. Data availability is a challenge — especially for fast-moving industries such as AI, in which data are often private or tied to proprietary information. For example, more data are needed on AI workloads in large cloud-computing companies, their electricity and carbon intensity, and trends in efficiencies gained for building and using AI models.
Methods to safely and openly share representative, measured, aggregated and anonymized data without compromising sensitive information are needed. AI can build on examples from other industries — such as the Getting the Numbers Right initiative, which keeps track of carbon dioxide and energy performance indicators in the global cement industry, and the Solomon Energy Intensity Index for fuel refining and pipelines.
Standards should be established for measuring, reporting, verifying and disseminating AI-related data, to ensure both quality and broad accessibility. Recent legislation, such as the European Union’s AI Act and the European Energy Efficiency Directive, could help to drive the development of standards. Although neither regulation directly mandates specific reporting on AI energy consumption, their emphasis on data-centre transparency and efficiency could promote the development of reporting standards.
Issue rapid updates. AI technology is advancing so quickly that scenarios will need to be revised at least once per year, and ideally twice. This is more frequent than is currently done for climate-change scenarios, which are updated every 6–7 years. Annual or biannual updates will be challenging, given the need to collect new data and to develop analytical frameworks as AI systems, applications and breakthroughs emerge.
Because of the potential for AI to either reduce or increase energy demand, researchers must update models that represent societal demand for energy, as well as explore how this demand will change as AI technologies evolve. Scenarios with varying resolutions might be released on different time frames. For example, coarse-resolution scenarios might be updated every few months; more-detailed scenarios could be released every 2–3 years.
Build an international consortium. An international consortium needs to be set up to undertake the development of AI-driven emissions scenarios. It should gather specialists from around the world and represent all the relevant disciplines — from computer and sustainability science to sociology and economics. We suggest this AI-driven emissions-scenario community be co-sponsored by international scientific networks that focus on sustainability, such as the International Institute for Applied Systems Analysis (IIASA) in Laxenburg, Austria, and by international non-governmental organizations focused on AI and society. Examples include the Partnership on AI or the newly established UN Futures Lab, which has been set up to coordinate and improve strategic foresight across the UN to guide long-term decision making.
Consortia that are associated with key IAM and energy-systems models, such as the IEA Technology Collaboration Programme or the IIASA’s programmes, could ensure both open access to data and models, and immediate relevance to the broader climate-scenario modelling communities. The UN and other bodies, such as the International Telecommunication Union in Geneva, Switzerland, should be engaged — but without compromising on the need for agility and speed.
Financial support will be needed to maintain the consortium and support the regular update of scenarios. This could come from a combination of philanthropic, private, governmental and intergovernmental sources.
AI is one of the most disruptive technologies of our time. It’s imperative that decisions around its development and use — today and as it evolves — are made with sustainability in mind. Only through developing a set of standard AI-driven emissions scenarios will policymakers, investors, advocates, private companies and the scientific community have the tools to make sound decisions regarding AI and the global race to net-zero emissions.
[ad_2]
Source Article Link