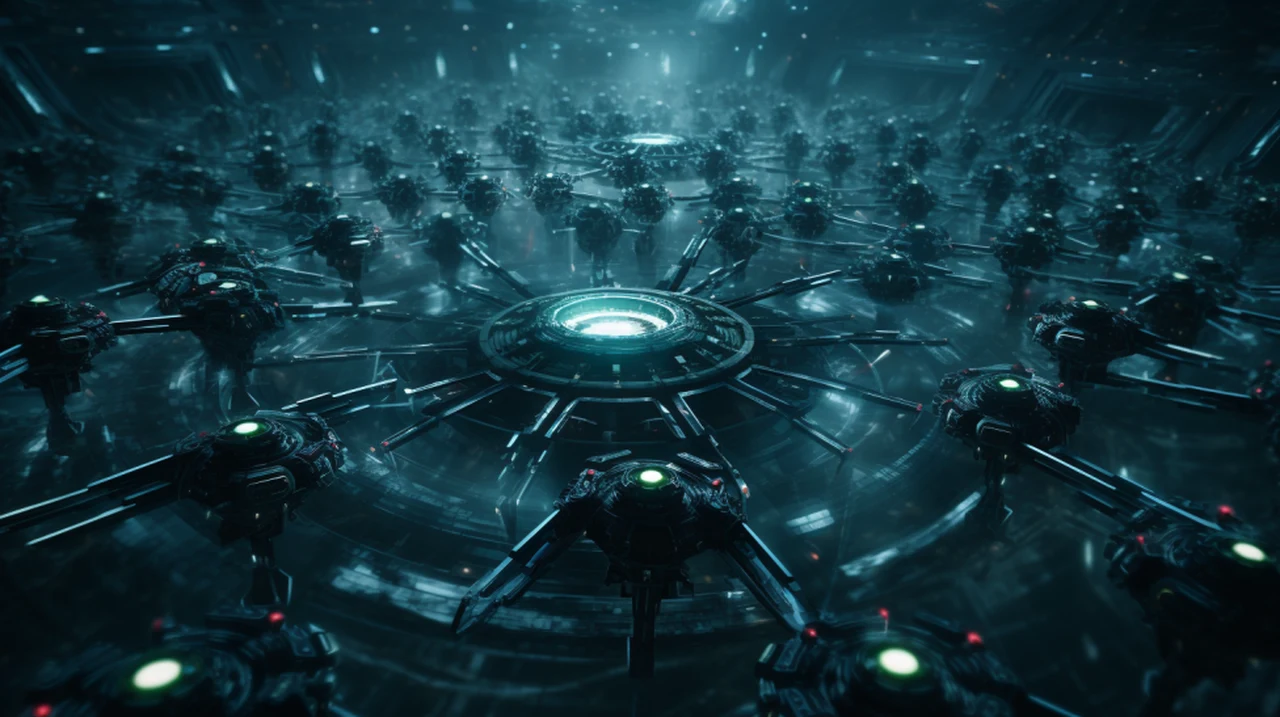
If you have been reading technology news websites recently you may have come across the term of AI agent swarms. But what are AI Agent Swarms and how are they important in the development of artificial intelligence? This quick overview guide will explain everything you need to know about AI swarms and how they can be used to improve workflow, productivity and save time. The first thing to remember is that these are coded AI models NOT physical drone style swarms which have starting to use liquid neural networks to control their navigation with precision.
First, let’s delve into what AI agent swarms are. In simple terms, these are groups of AI agents that work collaboratively to achieve specific goals. These agents, which are individual AI entities, operate in a coordinated manner, much like a flock of birds or a school of fish. The beauty of AI agent swarms lies in their collective behavior, which is more sophisticated and capable than the sum of its parts.
What are AI Agent Swarms?
To enhance your understanding, it’s important to know the core principles that govern AI agent swarms:
- Decentralization: Unlike traditional AI systems, there is no central control unit. Each agent in a swarm operates independently yet is interconnected with others.
- Scalability: Swarms can easily adapt to include more agents, enhancing their capabilities.
- Robustness: The decentralized nature makes these swarms resilient to failures. If one agent fails, others can compensate.
- Flexibility: These swarms can adapt to various environments and tasks, making them versatile in application.
Artificial Intelligence (AI) agents represent a significant stride in the realm of automation. These autonomous software entities, designed to execute tasks with minimal human intervention, range from simple data entry to complex problem-solving. When these agents operate in unison, they form an agent swarm – a collective intelligence adept at handling more substantial and intricate tasks.
These AI agents and swarms are not merely functional; they possess immense potential. Capable of interacting with digital environments like databases and Application Programming Interfaces (APIs), they excel at gathering and processing information. Additionally, they thrive in isolated environments through container technology, which ensures each agent performs its tasks efficiently and effectively without external interference.
Other articles we have written that you may find of interest on the subject of AI automation :
Beyond mere automation, AI agents are autonomous, capable of making decisions and adapting behaviors based on predefined goals or principles. This autonomy is a pivotal aspect of Artificial General Intelligence (AGI), a burgeoning concept that’s stirring conversations in mainstream media. AGI represents an AI capable of understanding, learning, and applying knowledge in diverse tasks akin to human abilities. The progression towards AGI is crucial for the evolution of fully autonomous agent swarms, which will revolutionize automation through advanced problem-solving and decision-making capabilities.
ACE Framework and the HOS Project
Two notable open-source initiatives propelling the advancement of autonomous AI are the Autonomous Cognitive Entity (ACE) framework and the Hierarchical Autonomous Agent Swarm (HOS) project. The ACE framework democratizes AI technology by leveraging open-source models and local hardware. It offers a cognitive architecture, a software blueprint for AI models, enabling developers to craft their AI agents. Conversely, the HOS project, utilizing the latest large language and multimodal models, facilitates the rapid creation of multiple agents in a cloud computing environment. This approach allows for scalable operations, significantly boosting the efficiency and effectiveness of AI applications.
The Autonomous AI Lab
Central to the development of these projects is the Autonomous AI Lab, a public Discord server that serves as a hub for collaboration on the ACE framework and the HOS project. This platform invites developers, researchers, and AI enthusiasts to engage in knowledge exchange, idea sharing, and collective development of autonomous AI technologies. The lab epitomizes the collaborative spirit necessary for advancing these cutting-edge projects, ensuring a future where AI agents and swarms play a pivotal role in various technological spheres.
Applications in Various Fields
AI agent swarms find applications in numerous domains, such as:
- Robotics: In drone swarms for agricultural monitoring or search and rescue operations.
- Data Analysis: They can process large datasets more efficiently by dividing the workload.
- Healthcare: For instance, in distributed diagnostics or patient care management.
The Mechanisms Behind Swarms
Now, in case you’re curious how these swarms work, let’s explore the mechanisms:
- Communication: Agents communicate with each other to share information and make decisions collaboratively.
- Self-organization: The ability to form organized patterns or behaviors without central control.
- Learning: Agents can learn from their environment and from other agents to improve their performance over time.
Challenges and Ethical Considerations
While AI agent swarms hold immense potential, they also present challenges:
- Control and Predictability: Ensuring that swarms behave as intended is complex.
- Security: They could be vulnerable to hacking or misuse.
- Ethical concerns: There are questions about accountability and decision-making processes in swarms.
How do AI agent swarms work?
AI agent swarms function through a blend of individual agent behaviors and collective dynamics, driven by algorithms and coding principles that mirror natural swarm behaviors like those of birds or insects. The coding of AI agent swarms involves several key aspects:
Each AI agent is an autonomous unit, programmed to perform specific tasks. These agents are usually coded with capabilities for decision-making, learning, and environmental interaction. This is achieved through various AI techniques like machine learning algorithms, rule-based systems, or a combination of both. The coding ensures that each agent can process inputs (like sensor data or communications from other agents), make decisions based on this data, and execute actions.
A critical aspect of swarm intelligence is the communication between agents. This is often facilitated through messaging protocols or shared environments. Agents exchange information about their status, observations, or decisions, allowing them to coordinate their actions. The communication code must be efficient and robust to ensure agents can synchronize their actions even in dynamic or unpredictable environments.
The most fascinating aspect of agent swarms is how simple rules at the individual level lead to complex collective behavior. Algorithms for collective behavior are inspired by nature and can include approaches like flocking, foraging, or collective exploration. These algorithms define how an agent reacts to the states or actions of its neighbors, leading to coordinated group behaviors like forming patterns, splitting, or merging.
To enhance the effectiveness of the swarm, agents often include adaptive algorithms allowing them to learn from their experiences and environment. This could involve reinforcement learning, where agents adjust their strategies based on feedback, or evolutionary algorithms that simulate natural selection processes to evolve more effective behaviors over time.
Coding AI swarms
Coding AI agent swarms involves developing individual agents with autonomous decision-making capabilities, implementing efficient communication protocols for inter-agent interactions, coding algorithms for collective behavior that drive the swarm’s intelligence, and incorporating adaptive and learning mechanisms. This blend of individual autonomy and collective coordination, driven by sophisticated coding, enables AI agent swarms to perform complex tasks more efficiently and robustly than single agents or traditional AI systems. The programming of these swarms is a complex task, requiring a deep understanding of both AI principles and the specific application domain.
AI agent swarms represent a cutting-edge development in the field of AI, offering remarkable possibilities for innovation and efficiency. Their decentralized, scalable, and robust nature, coupled with the ability to learn and self-organize, makes them an exciting area of study and application. As this technology continues to develop, it’s crucial to address the challenges and ethical considerations it brings. By understanding and harnessing the power of AI agent swarms, we can unlock new frontiers in various fields, driving forward the potential of artificial intelligence.
Filed Under: Guides, Top News
Latest timeswonderful Deals
Disclosure: Some of our articles include affiliate links. If you buy something through one of these links, timeswonderful may earn an affiliate commission. Learn about our Disclosure Policy.