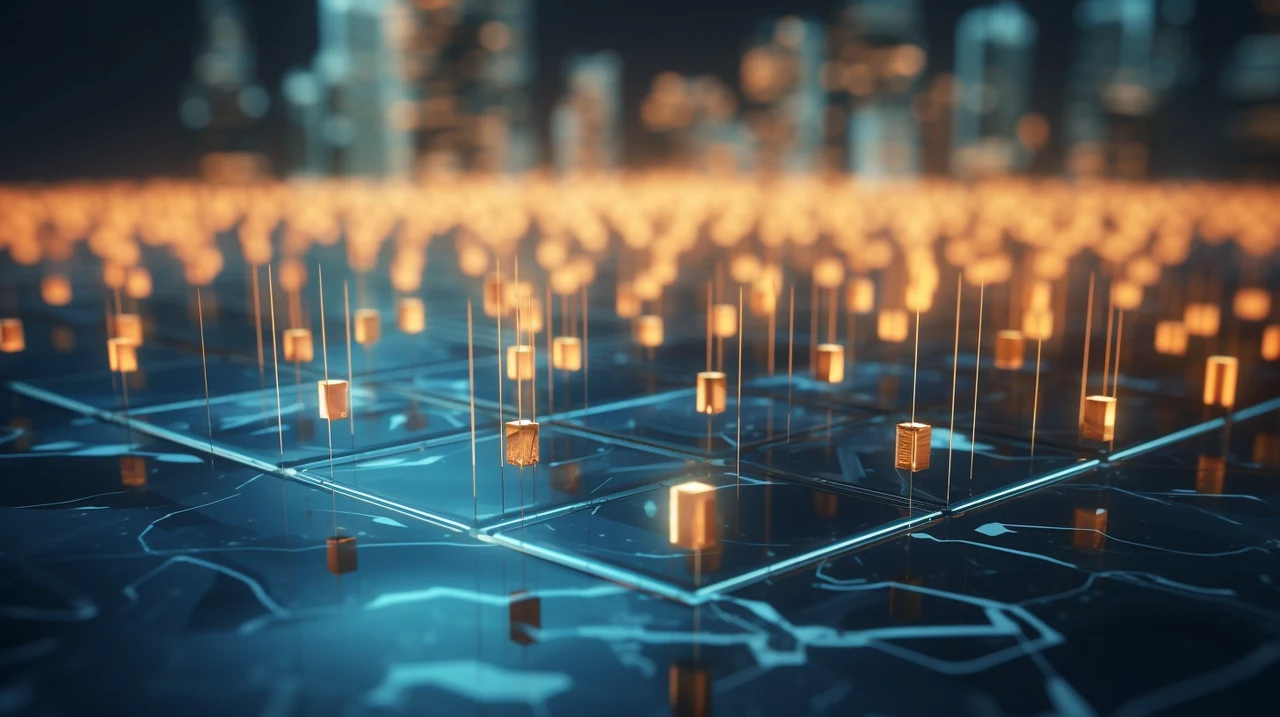
As artificial intelligence edges into every aspect of our life, it’s becoming clear that the broad capabilities of large language models (LLMs) like those from OpenAI aren’t always the perfect fit for every task. Instead, there’s a growing recognition of the value in creating specialized AI models that are fine-tuned to meet specific needs. These models offer a host of benefits, including enhanced speed, reduced costs, and greater predictability, which are not always achievable with one-size-fits-all solutions.
LLMs have made a significant impact with their advanced text processing and generation abilities, closely resembling human communication. However, when it comes to niche tasks, these models may fall short. They can be inefficient, lacking the speed or cost-effectiveness required for certain projects. Moreover, their general approach can lead to outputs that don’t have the necessary precision for specialized tasks. The Builder.io website has created a fantastic tutorial providing more insight into how you can train your own AI models.
Choosing to develop a custom AI model means you’re building a tool that aligns perfectly with the specific challenge you’re facing. This tailored approach can lead to more accurate and reliable results. Specialized models are also designed for efficiency, providing quick responses and saving valuable time. Another key benefit is cost efficiency; by focusing only on the features you need, you avoid paying for extras that don’t serve your purpose.
When you set out to create a specialized AI model, the first step is to break down your challenge into smaller, manageable pieces. This helps you understand the complexities of the task and identify the most effective AI strategies to employ. The next crucial step is to choose the right model type. The architecture of your AI model should match the specific data patterns and scenarios it will face, making this decision a cornerstone of the development process.
How to train and AI model with custom data
Here are some other articles you may find of interest on the subject of fine tuning AI :
Once you have a clear grasp of the problem and the model type you need, the next phase is to gather example data. This dataset should reflect the real-world situations your model will tackle and is essential for training the AI to respond accurately.
It’s also important to recognize the value of conventional programming. Sometimes, the best solutions come from a hybrid approach that combines traditional coding with AI models. Use conventional programming for parts of your problem that are deterministic, and apply AI for its predictive and flexible capabilities.
For those looking to streamline the development of their AI models, Google’s Vertex AI provides a user-friendly platform. It simplifies the process of training and deploying AI models, allowing you to manage them with minimal coding. Vertex AI supports a wide range of machine learning tasks, enabling you to focus on the unique aspects of your challenge.
Custom AI models
While LLMs have their place, a specialized AI model can often be a more fitting, efficient, and cost-effective choice for your specific needs. By methodically analyzing the problem, selecting the right model architecture, creating representative data, and blending traditional coding with AI where appropriate, you can create an AI solution that excels in addressing your particular demands. Tools like Google’s Vertex AI make this advanced capability more accessible, and the strategic combination of traditional coding and AI can unlock new problem-solving potential, leading to innovative and customized AI implementations.
The journey to developing a specialized AI model is both exciting and demanding. It requires a deep understanding of the problem at hand, a clear vision of the desired outcome, and a commitment to fine-tuning the model until it performs as needed. The process is iterative, involving testing, learning, and refining. But the rewards are substantial. A well-crafted AI model can provide insights and efficiencies that transform operations, drive innovation, and create competitive advantages.
AI specialization
As we continue to push the boundaries of what AI can do, the importance of specialization cannot be overstated. The ability to tailor AI models to specific tasks is not just a technical exercise; it’s a strategic imperative. It allows organizations to leverage the full power of AI in ways that are most relevant to their goals and challenges. Whether it’s improving customer service, optimizing supply chains, or advancing medical research, specialized AI models are becoming essential tools in the quest for excellence and innovation.
The development of these models is a collaborative effort, often involving teams of data scientists, engineers, and domain experts. Together, they work to ensure that the AI not only understands the data but also the context in which it operates. This collaboration is crucial because it ensures that the AI model is not just technically sound but also aligned with the real-world needs it is intended to serve.
As AI continues to evolve, the trend towards specialization is likely to grow. The demand for personalized, efficient, and cost-effective solutions is driving innovation in AI development, leading to more sophisticated and targeted models. These specialized models are not just tools for today; they are the building blocks for the intelligent systems of tomorrow.
For those looking to harness the power of AI, the message is clear: consider the unique aspects of your challenge and whether a specialized AI model could provide the solution you need. With the right approach and tools, the possibilities are virtually limitless. The future of AI is not just about more powerful models; it’s about smarter, more targeted solutions that deliver real value. And as we continue to explore the vast potential of AI, specialized models will play a pivotal role in turning that potential into reality.
Filed Under: Guides, Top News
Latest timeswonderful Deals
Disclosure: Some of our articles include affiliate links. If you buy something through one of these links, timeswonderful may earn an affiliate commission. Learn about our Disclosure Policy.