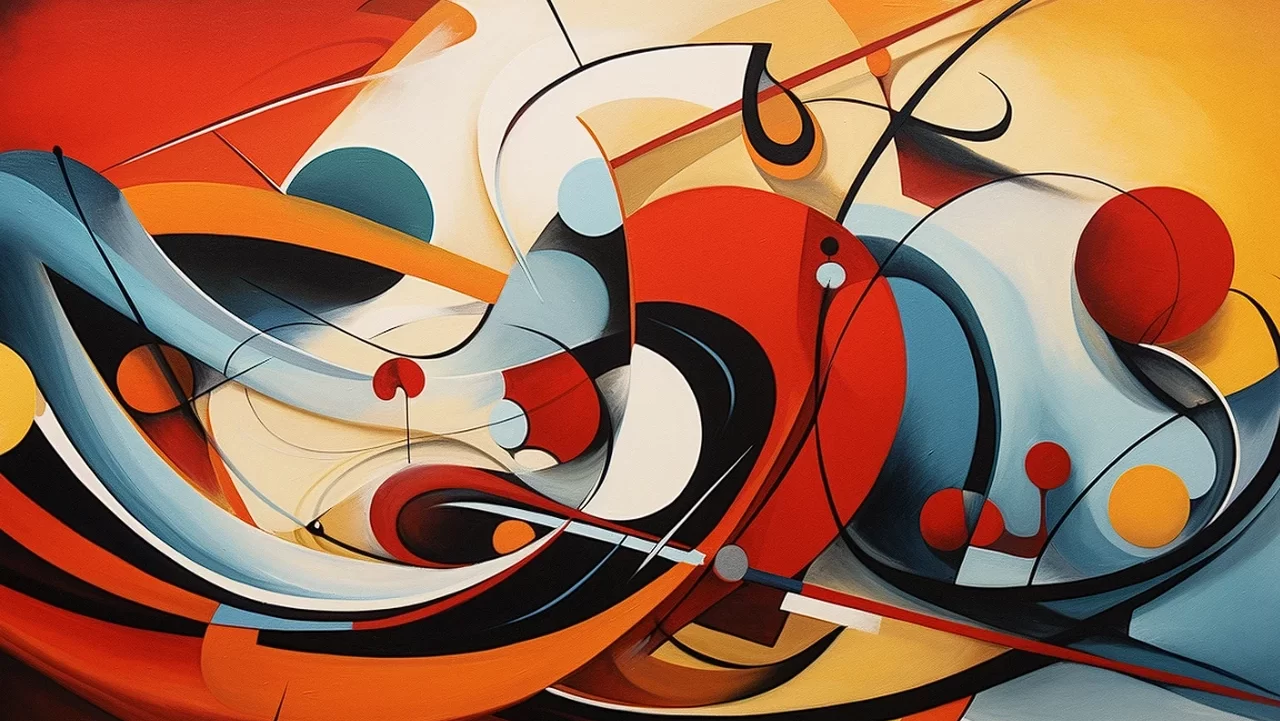
Artificial intelligence (AI) is transforming the way we interact with technology, but it’s not without its quirks. One such quirk is the phenomenon of AI hallucinations, where AI systems, particularly large language models like GPT-3 or BERT, sometimes generate responses that are incorrect or nonsensical. For those who rely on AI, it’s important to understand these issues to ensure the content produced by AI remains accurate and trustworthy. however these can be reduced by a number of techniques when fine-tuning AI models.”
AI hallucinations can occur for various reasons. Sometimes, they’re the result of adversarial attacks, where the AI is fed misleading data on purpose. More often, they happen by accident when the AI is trained on huge datasets that include errors or biases. The way these language models are built can also contribute to the problem.
To improve the reliability of AI outputs, there are several strategies you can use. One method is temperature prompting, which controls the AI’s creativity. Setting a lower temperature makes the AI’s responses more predictable and fact-based, while a higher temperature encourages creativity, which might not always be accurate.
Fine tuning AI models to reduce hallucinations
Imagine a world where your digital assistant not only understands you but also anticipates your needs with uncanny accuracy. This is the promise of advanced artificial intelligence (AI), but sometimes, this technology can lead to unexpected results. AI systems, especially sophisticated language models like GPT-3 or BERT, can sometimes produce what are known as “AI hallucinations.”
These are responses that may be incorrect, misleading, or just plain nonsensical. For users of AI technology, it’s crucial to recognize and address these hallucinations to maintain the accuracy and trustworthiness of AI-generated content. IBM provides more information on what you can consider when fine tuning AI models to reduce hallucinations.
Here are some other articles you may find of interest on the subject of fine-tuning artificial intelligence models and large language models LLMs :
The reasons behind AI hallucinations are varied. They can be caused by adversarial attacks, where harmful data is intentionally fed into the model to confuse it. More commonly, they occur unintentionally due to the training on large, unlabeled datasets that may contain errors and biases. The architecture of these language models, which are built as encoder-decoder models, also has inherent limitations that can lead to hallucinations.
To mitigate these issues, there are several techniques that can be applied to fine-tune your AI model, thus enhancing the reliability of its output. One such technique is temperature prompting, which involves setting a “temperature” parameter to manage the AI’s level of creativity. A lower temperature results in more predictable, factual responses, while a higher temperature encourages creativity at the expense of accuracy.
Another strategy is role assignment, where the AI is instructed to adopt a specific persona, such as a technical expert, to shape its responses to be more precise and technically sound. Providing the AI with detailed, accurate data and clear rules and examples, a method known as data specificity, improves its performance on tasks that demand precision, like scientific computations or coding.
Content grounding is another approach that anchors the AI’s responses in domain-specific information. Techniques like Retrieval Augmented Generation (RAG) help the AI pull data from a database to inform its responses, enhancing relevance and accuracy. Lastly, giving explicit instructions to the AI, outlining clear dos and don’ts, can prevent it from venturing into areas where it may offer unreliable information.
Fine tuning AI models
Another tactic is role assignment, where you tell the AI to act like a certain type of expert. This can help make its responses more accurate and technically correct. You can also give the AI more detailed data and clearer instructions, which helps it perform better on tasks that require precision, like math problems or programming.
Content grounding is another useful approach. It involves tying the AI’s responses to specific information from a certain field. For example, using techniques like Retrieval Augmented Generation (RAG) allows the AI to use data from a database to make its responses more relevant and correct.
Reducing hallucinations in AI models, particularly in large language models (LLMs) like GPT (Generative Pre-trained Transformer), is crucial for enhancing their reliability and trustworthiness. Hallucinations in AI context refer to instances where the model generates false or misleading information. This fine-tuning AI models guide outlines strategies and considerations for fine-tuning AI models to minimize these occurrences, focusing on both technical and ethical dimensions.
1. Understanding Hallucinations
Before attempting to mitigate hallucinations, it’s essential to understand their nature. Hallucinations can arise due to various factors, including but not limited to:
- Data Quality: Models trained on noisy, biased, or incorrect data may replicate these inaccuracies.
- Model Complexity: Highly complex models might overfit or generate outputs based on spurious correlations.
- Inadequate Context: LLMs might generate inappropriate responses if they misunderstand the context or lack sufficient information.
2. Data Curation and Enhancement
Improving the quality of the training data is the first step in reducing hallucinations.
- Data Cleaning: Remove or correct inaccurate, biased, or misleading content in the training dataset.
- Diverse Sources: Incorporate data from a wide range of sources to cover various perspectives and reduce bias.
- Relevance: Ensure the data is relevant to the model’s intended applications, emphasizing accuracy and reliability.
3. Model Architecture and Training Adjustments
Adjusting the model’s architecture and training process can also help minimize hallucinations.
- Regularization Techniques: Apply techniques like dropout or weight decay to prevent overfitting to the training data.
- Adversarial Training: Incorporate adversarial examples during training to improve the model’s robustness against misleading inputs.
- Dynamic Benchmarking: Regularly test the model against a benchmark dataset specifically designed to detect hallucinations.
4. Fine-tuning with High-Quality Data
Fine-tuning the pre-trained model on a curated dataset relevant to the specific application can significantly reduce hallucinations.
- Domain-Specific Data: Use high-quality, expert-verified datasets to fine-tune the model for specialized tasks.
- Continual Learning: Continuously update the model with new data to adapt to evolving information and contexts.
5. Prompt Engineering and Instruction Tuning
The way inputs (prompts) are structured can influence the model’s output significantly.
- Precise Prompts: Design prompts to clearly specify the type of information required, reducing ambiguity.
- Instruction Tuning: Fine-tune models using datasets of prompts and desired outputs to teach the model how to respond to instructions more accurately.
6. Post-Processing and Validation
Implementing post-processing checks can catch and correct hallucinations before the output is presented to the user.
- Fact-Checking: Use automated tools to verify factual claims in the model’s output against trusted sources.
- Output Filtering: Apply filters to detect and mitigate potentially harmful or nonsensical content.
7. Ethical Considerations and Transparency
- Disclosure: Clearly communicate the model’s limitations and the potential for inaccuracies to users.
- Ethical Guidelines: Develop and follow ethical guidelines for the use and deployment of AI models, considering their impact on individuals and society.
8. User Feedback Loop
Incorporate user feedback mechanisms to identify and correct hallucinations, improving the model iteratively.
- Feedback Collection: Allow users to report inaccuracies or hallucinations in the model’s output.
- Continuous Improvement: Use feedback to refine the data, model, and post-processing methods continuously.
By using these methods, you can enhance the user experience, fight the spread of misinformation, reduce legal risks, and build trust in AI models. As AI continues to evolve, it’s vital to ensure the integrity of these systems for them to be successfully integrated into our digital lives.
Implementing these fine tuning AI models strategies not only improves the user experience but also helps combat the spread of misinformation, mitigates legal risks, and fosters confidence in generative AI models. As AI technology progresses, ensuring the integrity of these models is crucial for their successful adoption in our increasingly digital world.
Filed Under: Guides, Top News
Latest timeswonderful Deals
Disclosure: Some of our articles include affiliate links. If you buy something through one of these links, timeswonderful may earn an affiliate commission. Learn about our Disclosure Policy.