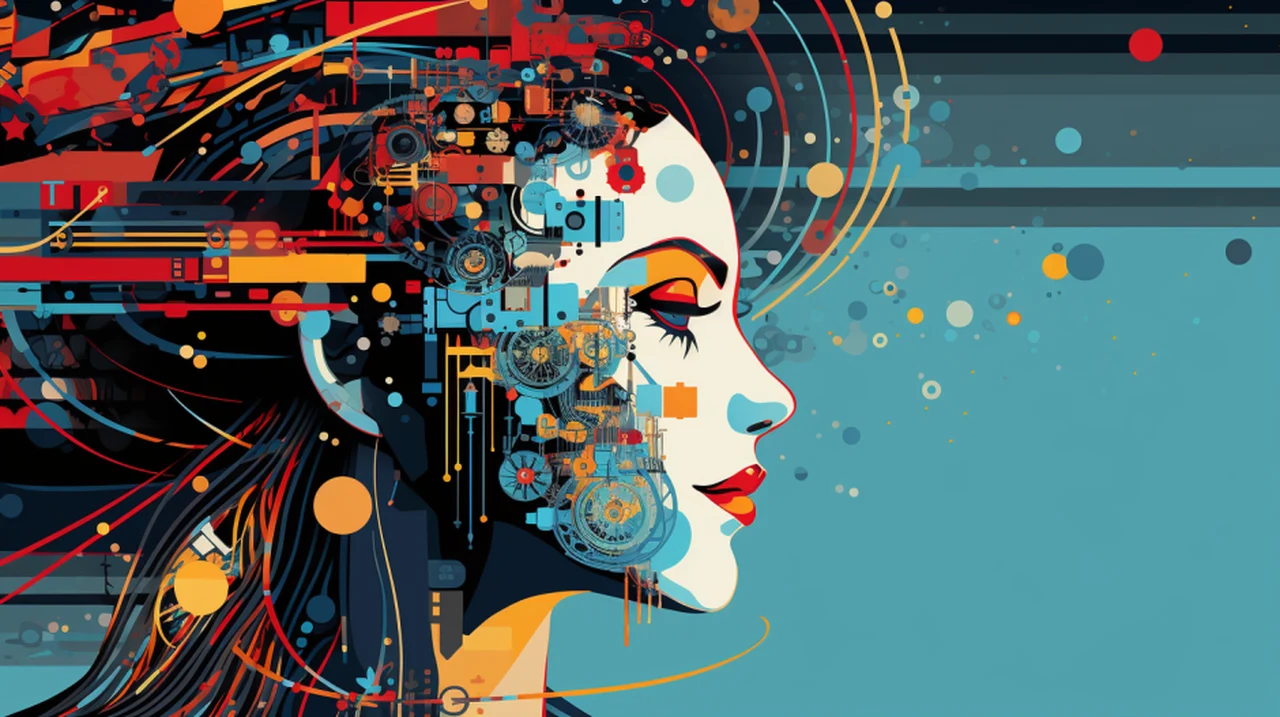
If you have engaged with the latest ChatGPT-4 AI model or perhaps the latest Google search engine, you will of already used multimodal artificial intelligence. However just a few years ago such easy access to multimodal AI was only a dream. In this guide will explain more about what this new technology is and how it is truly revolutionizing our world on a daily basis.
AI technologies that specialized in one form of data analysis, perhaps text-based chatbots or image recognition software is Single-Modality Learning . But now AI can combine different forms of data such as images, text, photographs, graphs, reports and more for a richer, more insightful analysis. These AI applications are multimodal AI in the already making their mark across many different areas of our lives.
For example in autonomous vehicles, multimodal AI helps in collecting data from cameras, LiDAR, and radar, combined it all for better situational awareness. In healthcare, AI can combine textual medical records with imaging data for more accurate diagnoses. In conversational agents such as ChatGPT-4, multimodal AI can interpret both the text and the tone of voice to provide more nuanced responses.
Multimodal Artificial Intelligence
- Single-Modality Learning: Handles only one type of input.
- Multimodal Learning: Can process multiple types of inputs like text, audio, and images.
Older machine learning models were unimodal, meaning they capable of only handling one type of input. For instance, text-based models like the Transformer architecture focus exclusively on textual data. Similarly, Convolutional Neural Networks (CNNs) are geared for visual data like images.
One area of multimodal AI technology you can try is within OpenAI’s ChatGPT. Now capable of interpreting inputs from text, files and imagery. Another is Google’s multimodal search engine. In essence, multimodal artificial intelligence (AI) systems are engineered to comprehend, interpret, and integrate multiple forms of data, be it text, images, audio, or even video. This versatile approach enhances the AI’s contextual understanding, thus making its outputs much more accurate.
What is Multimodal Artificial Intelligence?
The limitation here is evident—these models cannot naturally handle a mix of inputs, such as both audio and text. For example, you might have a conversational model that understands the text but fails to account for the tone or intonation captured in the audio, leading to misinterpretation.
In contrast, multimodal learning aims to build models that can process various types of inputs and possibly create a unified representation. This unification is beneficial because learning from one modality can enhance the model’s performance on another. Imagine a language model trained on both books and accompanying audiobooks; it might better understand the sentiment or context by aligning the text with the spoken words’ tone.
Another remarkable feature is the ability to generate common responses irrespective of the input type. In practical terms, this means the AI system could understand a query whether it’s typed in as text, spoken aloud, or even conveyed through a sequence of images. This has profound implications for accessibility, user experience, and the development of more robust systems. Let’s delve deeper into the facets of multimodal learning in machine learning models, a subfield that is garnering significant attention for its versatile applications and improved performance metrics. Key facets of multimodal AI include :
- Data Types: Includes text, images, audio, video, and more.
- Specialized Networks: Utilizes specialized neural networks like Convolutional Neural Networks (CNNs) for images and Recurrent Neural Networks (RNNs) or Transformers for text.
- Data Fusion: The integration of different data types through fusion techniques like concatenation, attention mechanisms, etc.
Simply put, integrating multiple data types allows for a more nuanced interpretation of complex situations. Imagine a healthcare scenario where a textual medical report might be ambiguous. Add to this X-ray images, and the AI system can arrive at a more definitive diagnosis. So, to enhance your experience with AI applications, multimodal systems offer a holistic picture by amalgamating disparate chunks of data.
In a multimodal architecture, different modules or neural networks are generally specialized for processing specific kinds of data. For example, a Convolutional Neural Network (CNN) might be used for image processing, while a Recurrent Neural Network (RNN) or Transformer might be employed for text. These specialized networks can then be combined through various fusion techniques, like concatenation, attention mechanisms, or more complex operations, to generate a unified representation.
In case you’re curious how these systems function, they often employ a blend of specialized networks designed for each data type. For instance, a CNN processes image data to extract relevant features, while a Transformer may process text data to comprehend its semantic meaning. These isolated features are then fused to create a holistic representation that captures the essence of the multifaceted input.
Fusion Techniques:
- Concatenation: Simply stringing together features from different modalities.
- Attention Mechanisms: Weighing the importance of features across modalities.
- Hybrid Architectures: More complex operations that dynamically integrate features during processing.
Simplified Analogies
he Orchestra Analogy: Think of multimodal AI as an orchestra. In a traditional, single-modal AI model, it’s as if you’re listening to just one instrument—say, a violin. That’s beautiful, but limited. With a multimodal approach, it’s like having an entire orchestra—violins, flutes, drums, and so on—playing in harmony. Each instrument (or data type) brings its unique sound (or insight), and when combined, they create a richer, fuller musical experience (or analysis).
The Swiss Army Knife Analogy: A traditional, single-modal AI model is like a knife with just one tool—a blade for cutting. Multimodal AI is like a Swiss Army knife, equipped with various tools for different tasks—scissors, screwdrivers, tweezers, etc. Just as you can tackle a wider range of problems with a Swiss Army knife, multimodal AI can handle more complex queries by utilizing multiple types of data.
Real-World Applications
To give you an idea of its vast potential, let’s delve into a few applications:
- Autonomous Vehicles: Sensor fusion leverages data from cameras, LiDAR, and radar to provide an exhaustive situational awareness.
- Healthcare: Textual medical records can be complemented by imaging data for a more thorough diagnosis.
- E-commerce: Recommender systems can incorporate user text reviews and product images for enhanced recommendations.
Google, with its multimodal capabilities in search algorithms, leverages both text and images to give you a more complete set of search results. Similarly, Tesla excels in implementing multimodal sensor fusion in its self-driving cars, capturing a 360-degree view of the car’s surroundings.
The importance of multimodal learning primarily lies in its ability to generate common representations across diverse inputs. For instance, in a healthcare application, a multimodal model might align a patient’s verbal description of symptoms with medical imaging data to provide a more accurate diagnosis. These aligned representations enable the model to understand the subject matter more holistically, leveraging complementary information from different modalities for a more rounded view.
Multimodal AI has immense promise but is also subject to ongoing research to solve challenges like data alignment and modality imbalance. However, with advancements in deep learning and data science, this field is poised for significant growth.
So there you have it, a sweeping yet accessible view of what multimodal AI entails. With the ability to integrate a medley of data types, this technology promises a future where AI is not just smart but also insightful and contextually aware.
Multimodal Artificial Intelligence (AI) summary:
- Single-Modality Learning: Handles only one type of input.
- Multimodal Learning: Can process multiple types of inputs like text, audio, and images.
- Cross-Modality Benefits: Learning from one modality can enhance performance in another.
- Common Responses: Capable of generating unified outputs irrespective of input type.
- Common Representations: Central to the multimodal approach, allowing for a holistic understanding of diverse data types.
Multimodal learning offers an evolved, nuanced approach to machine learning. By fostering common representations across a spectrum of inputs, these models are pushing the boundaries of what AI can perceive, interpret, and act upon.
Filed Under: Guides, Top News
Latest timeswonderful Deals
Disclosure: Some of our articles include affiliate links. If you buy something through one of these links, timeswonderful may earn an affiliate commission. Learn about our Disclosure Policy.