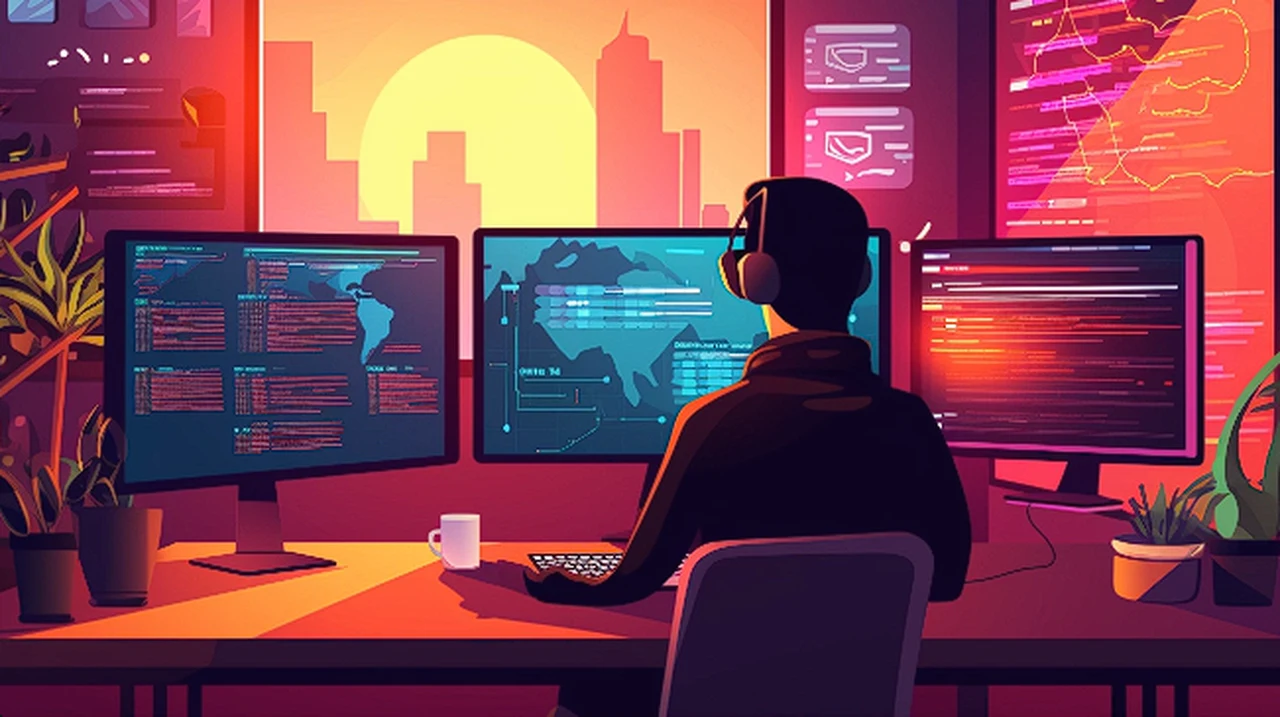
With artificial intelligence (AI) exploding into our lives this year more than ever before you might be interested to know a little more about the technologies that have been used to create many of the AI tools and services that are currently being developed and released in early development. The world of AI is a fascinating place and features lots of new technologies in terms that we are trying to get to grips with. This guide will provide more information on the differences between Machine Learning vs Deep Learning.
At its core, machine learning is a subset of AI that enables software applications to predict outcomes more accurately without being explicitly programmed to do so. It’s the art of giving computers the ability to learn from data, identify patterns, and make decisions with minimal human intervention. Machine learning algorithms can handle historical data as input to predict new output values. This encompasses various types, including supervised, unsupervised, and reinforcement learning.
Machine Learning vs Deep Learning
Simplifying the differences
- Definition:
- Machine Learning is a subset of AI that enables machines to improve at tasks with experience.
- Deep Learning is a subset of Machine Learning that uses layered neural networks to simulate human decision-making.
- Approach:
- Machine Learning algorithms often require structured data to learn and make predictions.
- Deep Learning algorithms learn from data that is often unstructured and high-dimensional, like images and audio.
- Complexity:
- Machine Learning models are generally simpler and can work on traditional CPUs.
- Deep Learning models are more complex, involving many layers in neural networks, and usually require GPUs for computation.
- Data Requirements:
- Machine Learning can work with smaller datasets and still perform well.
- Deep Learning requires large amounts of data to understand and learn effectively.
- Performance:
- Machine Learning models may plateau on performance as more data is fed in.
- Deep Learning models tend to improve their performance with more data and complexity.
- Usage Scenarios:
- Machine Learning is suitable for tasks like spam detection, simple recommendation systems, and predictive analytics.
- Deep Learning excels at more complex tasks like image recognition, speech recognition, and natural language processing.
- Interpretability:
- Machine Learning models are often easier to interpret and understand.
- Deep Learning models, due to their complexity, are typically considered “black boxes” with lower interpretability.
Deep Learning, a subset of machine learning, takes inspiration from the human brain. Here, artificial neural networks, which mimic the way neurons signal each other, are used to process data in complex ways. These neural networks have multiple layers that can learn increasingly abstract concepts, allowing DL algorithms to handle unstructured data such as images and text more effectively than traditional Machine Learning algorithms.
The difference between Machine Learning vs Deep Learning can be intriguing. Deep learning algorithms are generally more complex, requiring a deeper architecture compared to their machine learning counterparts. While machine learning can work with smaller datasets, deep learning requires a large volume of data to perform optimally. In terms of hardware, DL often relies on high-end GPUs due to its higher computational power demands. As for application scope, machine learning is suitable for problems with limited data and computational resources, whereas deep learning excels at tasks that involve massive amounts of data.
Machine learning in action
Machine learning is a transformative technology, an innovation that fundamentally changes existing processes, habits, or industries in a significant and often disruptive way. Making a significant impact on our everyday digital experience, often in ways we might not immediately recognize. Let’s delve into two of the most ubiquitous applications of machine learning: email filtering and recommendation systems.
Email Filtering Systems
Email filtering is a critical function that most of us benefit from every time we open our inbox. Here’s how machine learning contributes to this process:
- Spam Detection: Machine learning models are trained to distinguish between spam and non-spam by learning from vast quantities of labeled data. These models look for specific patterns that are commonly found in spam emails, such as certain keywords, sender’s email addresses, or even the formatting of the email.
- User Behavior: Over time, these algorithms adapt to the individual user’s behavior. If a user frequently marks messages from a particular sender as spam, the ML system learns to automatically filter similar messages in the future.
- Continuous Learning: The beauty of machine learning in email filtering is its ability to continuously learn and adapt. As spammers evolve their tactics, the machine learning models keep up by learning from the new patterns that emerge.
Recommendation Systems
Recommendation systems are another area where machine learning shines, particularly in streaming platforms like Netflix. Here’s how they work:
- Personalized Suggestions: Machine learning algorithms analyze your viewing history to make personalized movie or show recommendations. They use complex algorithms to find patterns in your choices and compare them with other users who have similar tastes.
- Content Attributes: These systems also examine the attributes of the films and shows you watch, including genres, actors, and even the directors, to find and suggest content with similar characteristics.
- Improving Engagement: The goal is to keep you engaged with the platform by effectively predicting what you might enjoy watching next. A well-tuned recommendation system can be a key differentiator for a service like Netflix in retaining its user base.
Both these applications are clear examples of machine learning’s capacity to enhance user experience in very practical and impactful ways. By harnessing the power of ML, services can provide a level of personalization and efficiency that simply wasn’t possible before.
Deep learning driving innovation
Deep learning, with its advanced capabilities in handling intricate tasks, is indeed revolutionizing sectors where traditional machine learning techniques may fall short. Let’s delve deeper into how deep learning propels innovations in autonomous vehicles and voice assistants.
Autonomous Vehicles
In the realm of autonomous vehicles, deep learning plays a pivotal role, especially in the following aspects:
- Computer Vision: Deep learning models, through convolutional neural networks (CNNs), enable vehicles to interpret visual information from cameras. These networks are adept at processing and analyzing images to recognize traffic signs, pedestrians, other vehicles, and road markings.
- Sensor Fusion: Deep learning algorithms can integrate data from various sensors such as LIDAR, radar, and cameras to create a comprehensive understanding of the vehicle’s surroundings, a process known as sensor fusion. This is critical for safe navigation and real-time decision-making.
- Predictive Analytics: Deep learning also helps in predictive analytics, where the vehicle can anticipate potential hazards or the behavior of other road users. This predictive capacity is vital for the proactive safety measures required in autonomous driving.
Voice Assistants
For voice assistants like Siri and Alexa, deep learning has brought about significant improvements:
- Natural Language Processing (NLP): Deep learning models, particularly recurrent neural networks (RNNs) and transformers, have greatly advanced the field of NLP. They enable voice assistants to understand and generate human language with a level of fluency that is increasingly natural and responsive.
- Speech Recognition: Voice assistants are becoming more adept at accurately transcribing spoken words into text, thanks to deep neural networks that can capture the nuances of human speech, including accents and intonation.
- Contextual Understanding: Beyond recognizing words, deep learning allows these assistants to grasp the context of a conversation. This capability means they can handle follow-up questions, remember user preferences, and even detect subtleties like sarcasm or implied meaning.
Enhancing Reliability and Interactivity
The advanced capabilities of deep learning are not just making these technologies possible but are also enhancing their reliability and interactivity. Autonomous vehicles are becoming safer and closer to widespread adoption. At the same time, voice assistants are transitioning from being simple command-based interfaces to more interactive and engaging companions capable of carrying out complex tasks.
Other articles you may find of interest on the subject of Deep Learning and Machine Learning :
The future of AI
Deep learning serves as the backbone of some of the most cutting-edge technologies today. Its ability to process and learn from enormous datasets is what enables machines to perform tasks that require a level of understanding and decision-making that was once thought to be exclusively human.
The technical depth of Machine Learning vs Deep Learning can be overwhelming, but at their core, these technologies are built on a few fundamental principles. Both use algorithms, which are sets of rules and statistical techniques to analyze and interpret data. Training a model on a dataset to perform a specific task, such as recognizing speech or classifying images, is a cornerstone of both Machine Learning and Deep Learning.
With the continuous evolution of these technologies, one can’t help but be excited about the potential advancements they promise. Companies like Google invest heavily in both Machine Learning vs Deep Learning to enhance their products and services. Whatever technology used the goal is to create systems that can learn and adapt—just like we do.
Machine learning is an exceptional tool for data analysis and prediction, well-suited for less complex tasks. Deep learning, on the other hand, elevates this capability, allowing machines to perform highly complex tasks by emulating the intricate workings of the human brain. Both Machine Learning and Deep Learning are driving us towards a future where technology seamlessly integrates into our daily lives, simplifying tasks, and unlocking new possibilities. As you delve deeper into these domains, remember the balance between data, computational requirements, and the task’s complexity is key to finding the right technological solution for your needs.
Filed Under: Guides, Top News
Latest timeswonderful Deals
Disclosure: Some of our articles include affiliate links. If you buy something through one of these links, timeswonderful may earn an affiliate commission. Learn about our Disclosure Policy.