[ad_1]
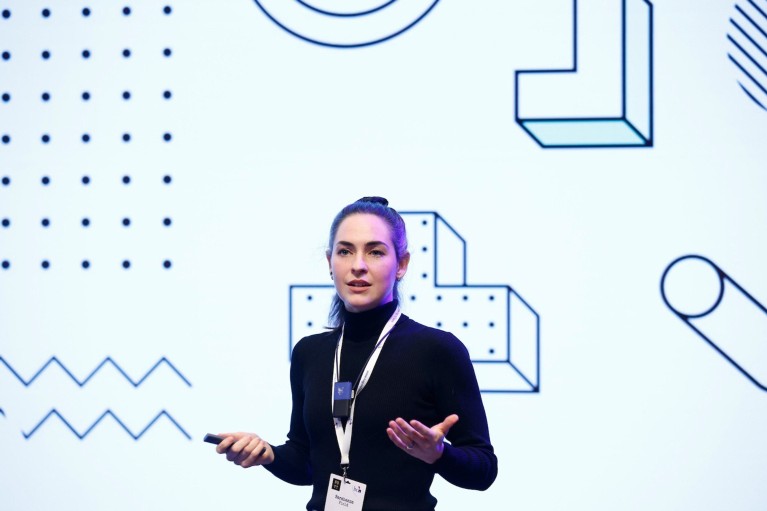
The editor-in-chief of the Journal of Trial & Error, Sarahanne Field wants to publish the messy, null and negative results sitting in researchers’ file drawers.Credit: Sander Martens
Editor-in-chief Sarahanne Field describes herself and her team at the Journal of Trial & Error as wanting to highlight the “ugly side of science — the parts of the process that have gone wrong”.
She clarifies that the editorial board of the journal, which launched in 2020, isn’t interested in papers in which “you did a shitty study and you found nothing. We’re interested in stuff that was done methodologically soundly, but still yielded a result that was unexpected.” These types of result — which do not prove a hypothesis or could yield unexplained outcomes — often simply go unpublished, explains Field, who is also an open-science researcher at the University of Groningen in the Netherlands. Along with Stefan Gaillard, one of the journal’s founders, she hopes to change that.
Calls for researchers to publish failed studies are not new. The ‘file-drawer problem’ — the stacks of unpublished, negative results that most researchers accumulate — was first described in 1979 by psychologist Robert Rosenthal. He argued that this leads to publication bias in the scientific record: the gap of missing unsuccessful results leads to overemphasis on the positive results that do get published.
Careers Collection: Publishing
Over the past 30 years, the proportion of negative results being published has decreased further. A 2012 study showed that, from 1990 to 2007, there was a 22% increase in positive conclusions in papers; by 2007, 85% of papers published had positive results1. “People fail to report [negative] results, because they know they won’t get published — and when people do attempt to publish them, they get rejected,” says Field. A 2022 survey of researchers in France in chemistry, physics, engineering and environmental sciences showed that, although 81% had produced relevant negative results and 75% were willing to publish them, only 12.5% had the opportunity to do so2.
One factor that is leading some researchers to revisit the problem is the growing use of predictive modelling using machine-learning tools in many fields. These tools are trained on large data sets that are often derived from published work, and scientists have found that the absence of negative data in the literature is hampering the process. Without a concerted effort to publish more negative results that artificial intelligence (AI) can be trained on, the promise of the technology could be stifled.
“Machine learning is changing how we think about data,” says chemist Keisuke Takahashi at Hokkaido University in Japan, who has brought the issue to the attention of the catalysis-research community. Scientists in the field have typically relied on a mixture of trial and error and serendipity in their experiments, but there is hope that AI could provide a new route for catalyst discovery. Takahashi and his colleagues mined data from 1,866 previous studies and patents to train a machine-learning model to predict the best catalyst for the reaction between methane and oxygen to form ethane and ethylene, both of which are important chemicals used in industry3. But, he says, “over the years, people have only collected the good data — if they fail, they don’t report it”. This led to a skewed model that, in some cases, enhanced the predicted performance of a material, rather than realistically assessing its properties.
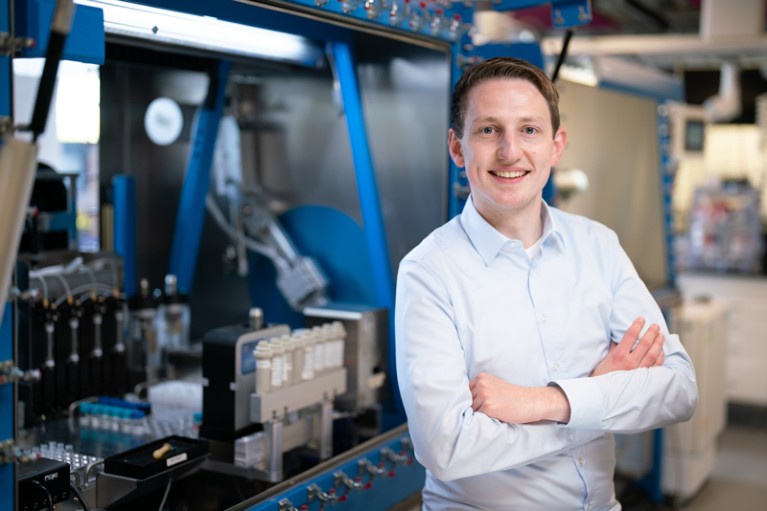
Synthetic organic chemist Felix Strieth-Kalthoff found that published data were too heavily biased toward positive results to effectively train an AI model to optimize chemical reaction yields.Credit: Cindy Huang
Alongside the flawed training of AI models, the huge gap of negative results in the scientific record continues to be a problem across all disciplines. In areas such as psychology and medicine, publication bias is one factor exacerbating the ongoing reproducibility crisis — in which many published studies are impossible to replicate. Without sharing negative studies and data, researchers could be doomed to repeat work that led nowhere. Many scientists are calling for changes in academic culture and practice — be it the creation of repositories that include positive and negative data, new publication formats or conferences aimed at discussing failure. The solutions are varied, but the message is the same: “To convey an accurate picture of the scientific process, then at least one of the components should be communicating all the results, [including] some negative results,” says Gaillard, “and even where you don’t end up with results, where it just goes wrong.”
Science’s messy side
Synthetic organic chemist Felix Strieth-Kalthoff, who is now setting up his own laboratory at the University of Wuppertal, Germany, has encountered positive-result bias when using data-driven approaches to optimize the yields of certain medicinal-chemistry reactions. His PhD work with chemist Frank Glorius at the University of Münster, Germany, involved creating models that could predict which reactants and conditions would maximize yields. Initially, he relied on data sets that he had generated from high-throughput experiments in the lab, which included results from both high- and low-yield reactions, to train his AI model. “Our next logical step was to do that based on the literature,” says Strieth-Kalthoff. This would allow him to curate a much larger data set to be used for training.
But when he incorporated real data from the reactions database Reaxys into the training process, he says, “[it] turned out they don’t really work at all”. Strieth-Kalthoff concluded the errors were due the lack of low-yield reactions4; “All of the data that we see in the literature have average yields of 60–80%.” Without learning from the messy ‘failed’ experiments with low yields that were present in the initial real-life data, the AI could not model realistic reaction outcomes.
Although AI has the potential to spot relationships in complex data that a researcher might not see, encountering negative results can give experimentalists a gut feeling, says molecular modeller Berend Smit at the Swiss Federal Institute of Technology Lausanne. The usual failures that every chemist experiences at the bench give them a ‘chemical intuition’ that AI models trained only on successful data lack.
Smit and his team attempted to embed something similar to this human intuition into a model tasked with designing a metal-organic framework (MOF) with the largest known surface area for this type of material. A large surface area allows these porous materials to be used as reaction supports or molecular storage reservoirs. “If the binding [between components] is too strong, it becomes amorphous; if the binding is too weak, it becomes unstable, so you need to find the sweet spot,” Smit says. He showed that training the machine-learning model on both successful and unsuccessful reaction conditions created better predictions and ultimately led to one that successfully optimized the MOF5. “When we saw the results, we thought, ‘Wow, this is the chemical intuition we’re talking about!’” he says.
According to Strieth-Kalthoff, AI models are currently limited because “the data that are out there just do not reflect all of our knowledge”. Some researchers have sought statistical solutions to fill the negative-data gap. Techniques include oversampling, which means supplementing data with several copies of existing negative data or creating artificial data points, for example by including reactions with a yield of zero. But, he says, these types of approach can introduce their own biases.
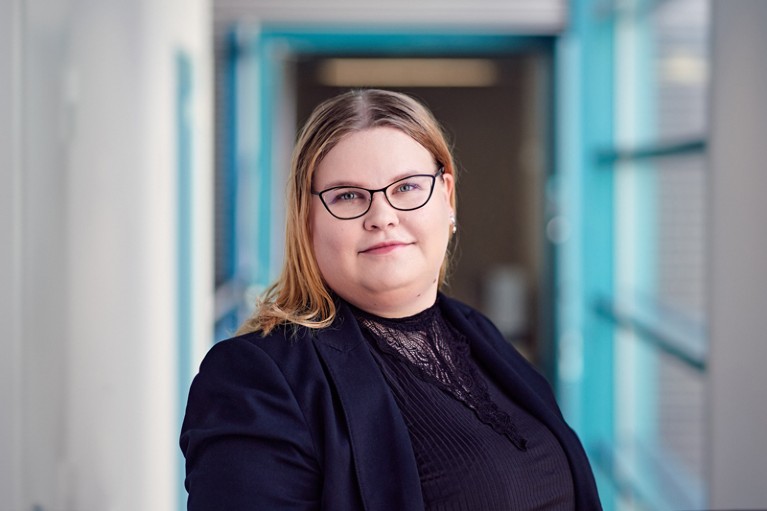
Computer scientist Ella Peltonen helped to organize the first International Workshop on Negative Results in Pervasive Computing in 2022 to give researchers an opportunity to discuss failed experiments.Credit: University of Oulu
Capturing more negative data is now a priority for Takahashi. “We definitely need some sort of infrastructure to share the data freely.” His group has created a website for sharing large amounts of experimental data for catalysis reactions. Other organizations are trying to collect and publish negative data — but Takahashi says that, so far, they lack coordination, so data formats aren’t standardized. In his field, Strieth-Kalthoff says, there are initiatives such as the Open Reaction Database, launched in 2021 to share organic-reaction data and enable training of machine-learning applications. But, he says, “right now, nobody’s using it, [because] there’s no incentive”.
Smit has argued for a modular open-science platform that would directly link to electronic lab notebooks to help to make different data types extractable and reusable. Through this process, publication of negative data in peer-reviewed journals could be skipped, but the information would still be available for researchers to use in AI training. Strieth-Kalthoff agrees with this strategy in theory, but thinks it’s a long way off in practice, because it would require analytical instruments to be coupled to a third-party source to automatically collect data — which instrument manufacturers might not agree to, he says.
Publishing the non-positive
In other disciplines, the emphasis is still on peer-reviewed journals that will publish negative results. Gaillard, a science-studies PhD student at Radboud University in Nijmegen, the Netherlands, co-founded the Journal of Trial & Error after attending talks on how science can be made more open. Gaillard says that, although everyone whom they approached liked the idea of the journal, nobody wanted to submit articles at first. He and the founding editorial team embarked on a campaign involving cold calls and publicity at open-science conferences. “Slowly, we started getting our first submissions, and now we just get people sending things in [unsolicited],” he says. Most years the journal publishes one issue of about 8–14 articles, and it is starting to publish more special issues. It focuses mainly on the life sciences and data-based social sciences.
In 2008, David Alcantara, then a chemistry PhD student at the University of Seville in Spain who was frustrated by the lack of platforms for sharing negative results, set up The All Results journals, which were aimed at disseminating results regardless of the outcome. Of the four disciplines included at launch, only the biology journal is still being published. “Attracting submissions has always posed a challenge,” says Alcantara, now president at the consultancy and training organization the Society for the Improvement of Science in Seville.
But Alcantara thinks there has been a shift in attitudes: “More established journals [are] becoming increasingly open to considering negative results for publication.” Gaillard agrees: “I’ve seen more and more journals, like PLoS ONE, for example, that explicitly mentioned that they also publish negative results.” (Nature welcomes submissions of replication studies and those that include null results, as described in this 2020 editorial.)
Journals might be changing their publication preferences, but there are still significant disincentives that stop researchers from publishing their file-drawer studies. “The current academic system often prioritizes high-impact publications and ground-breaking discoveries for career advancement, grants and tenure,” says Alcantara, noting that negative results are perceived as contributing little to nothing to these endeavours. Plus, there is still a stigma associated with any kind of failure. “People are afraid that this will look negative on their CV,” says Gaillard. Smit describes reporting failed experiments as a no-win situation: “It’s more work for [researchers], and they don’t get anything in return in the short term.” And, jokes Smit, what’s worse is that they could be providing data for an AI tool to take over their role.
Ultimately, most researchers conclude that publishing their failed studies and negative data is just not worth the time and effort — and there’s evidence that they judge others’ negative research more harshly than positive outcomes. In a study published in August, 500 researchers from top economics departments around the world were randomized to two groups and asked to judge a hypothetical research paper. Half of the participants were told that the study had a null conclusion, and the other half were told the results were sizeably significant. The null results were perceived to be 25% less likely to be published, of lower quality and less important than were the statistically significant findings6.
Some researchers have had positive experiences sharing their unsuccessful findings. For example, in 2021, psychologist Wendy Ross at the London Metropolitan University published her negative results from testing a hypothesis about human problem-solving in the Journal of Trial & Error7, and says the paper was “the best one I have published to date”. She adds, “Understanding the reasons for null results can really test and expand our theoretical understanding.”
Fields forging solutions
The field of psychology has introduced one innovation that could change publication biases — registered reports (RRs). These peer-reviewed reports, first published in 2014, came about largely as a response to psychology’s replication crisis, which began in around 2011. RRs set out the methodology of a study before the results are known, to try to prevent selective reporting of positive results. Daniël Lakens, who studies science-reward structures at Eindhoven University of Technology in the Netherlands, says there is evidence that RRs increase the proportion of negative results in the psychology literature.
In a 2021 study, Lakens analysed the proportion of published RRs whose results eventually support the primary hypothesis. In a random sample of hypothesis-testing studies from the standard psychology literature, 96% of the results were positive. In RRs, this fell to only 44%8. Lakens says the study shows “that if you offer this as an option, many more null results enter the scientific literature, and that is a desirable thing”. At least 300 journals, including Nature, are now accepting RRs, and the format is spreading to journals in biology, medicine and some social-science fields.
Yet another approach has emerged from the field of pervasive computing, the study of how computer systems are integrated into physical surroundings and everyday life. About four years ago, members of the community started discussing reproducibility, says computer scientist Ella Peltonen at the University of Oulu in Finland. Peltonen says that researchers realized that, to avoid the repetition of mistakes, there was a need to discuss the practical problems with studies and failed results that don’t get published. So in 2022, Peltonen and her colleagues held the first virtual International Workshop on Negative Results in Pervasive Computing (PerFail), in conjunction with the field’s annual conference, the International Conference on Pervasive Computing and Communications.
Peltonen explains that PerFail speakers first present their negative results and then have the same amount of time for discussion afterwards, during which participants tease out how failed studies can inform future work. “It also encourages the community to showcase that things require effort and trial and error, and there is value in that,” she adds. Now an annual event, the organizers invite students to attend so they can see that failure is a part of research and that “you are not a bad researcher because you fail”, says Peltonen.
In the long run, Alcantara thinks a continued effort to persuade scientists to share all their results needs to be coupled with policies at funding agencies and journals that reward full transparency. “Criteria for grants, promotions and tenure should recognize the value of comprehensive research dissemination, including failures and negative outcomes,” he says. Lakens thinks funders could be key to boosting the RR format, as well. Funders, he adds, should say, “We want the research that we’re funding to appear in the scientific literature, regardless of the significance of the finding.”
There are some positive signs of change about sharing negative data: “Early-career researchers and the next generation of scientists are particularly receptive to the idea,” says Alcantara. Gaillard is also optimistic, given the increased interest in his journal, including submissions for an upcoming special issue on mistakes in the medical domain. “It is slow, of course, but science is a bit slow.”
[ad_2]
Source Article Link