[ad_1]
Hello Nature readers, would you like to get this Briefing in your inbox free every week? Sign up here.
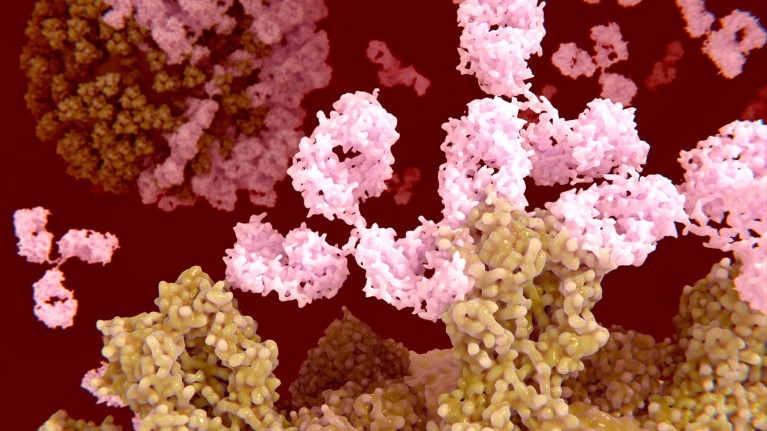
Credit: Juan Gaertner/Science Photo Library
For the first time, an AI system has helped researchers to design completely new antibodies. An algorithm similar to those of the image-generating tools Midjourney and DALL·E has churned out thousands of new antibodies that recognize certain bacterial, viral or cancer-related targets. Although in laboratory tests only about one in 100 designs worked as hoped, biochemist and study co-author Joseph Watson says that “it feels like quite a landmark moment”.
Reference: bioRxiv preprint (not peer reviewed)
US computer-chip giant Nvidia says that a ‘superchip’ made up of two of its new ‘Blackwell’ graphics processing units and its central processing unit (CPU), offers 30 times better performance for running chatbots such as ChatGPT than its previous ‘Hopper’ chips — while using 25 times less energy. The chip is likely to be so expensive that it “will only be accessible to a select few organisations and countries”, says Sasha Luccioni from the AI company Hugging Face.
A machine-learning tool shows promise for detecting COVID-19 and tuberculosis from a person’s cough. While previous tools used medically annotated data, this model was trained on more than 300 million clips of coughing, breathing and throat clearing from YouTube videos. Although it’s too early to tell whether this will become a commercial product, “there’s an immense potential not only for diagnosis, but also for screening” and monitoring, says laryngologist Yael Bensoussan.
Reference: arXiv preprint (not peer reviewed)
In blind tests, five football experts favoured an AI coach’s corner-kick tactics over existing ones 90% of the time. ‘TacticAI’ was trained on more than 7,000 examples of corner kicks provided by the UK’s Liverpool Football Club. These are major opportunities for goals and strategies are determined ahead of matches. “What’s exciting about it from an AI perspective is that football is a very dynamic game with lots of unobserved factors that influence outcomes,” says computer scientist and study co-author Petar Veličković.
Reference: Nature Communications paper
Features & opinion
AI image generators can amplify biased stereotypes in their output. There have been attempts to quash the problem by manual fine-tuning (which can have unintended consequences, for example generating diverse but historically inaccurate images) and by increasing the amount of training data. “People often claim that scale cancels out noise,” says cognitive scientist Abeba Birhane. “In fact, the good and the bad don’t balance out.” The most important step to understanding how these biases arise and how to avoid them is transparency, researchers say. “If a lot of the data sets are not open source, we don’t even know what problems exist,” says Birhane.
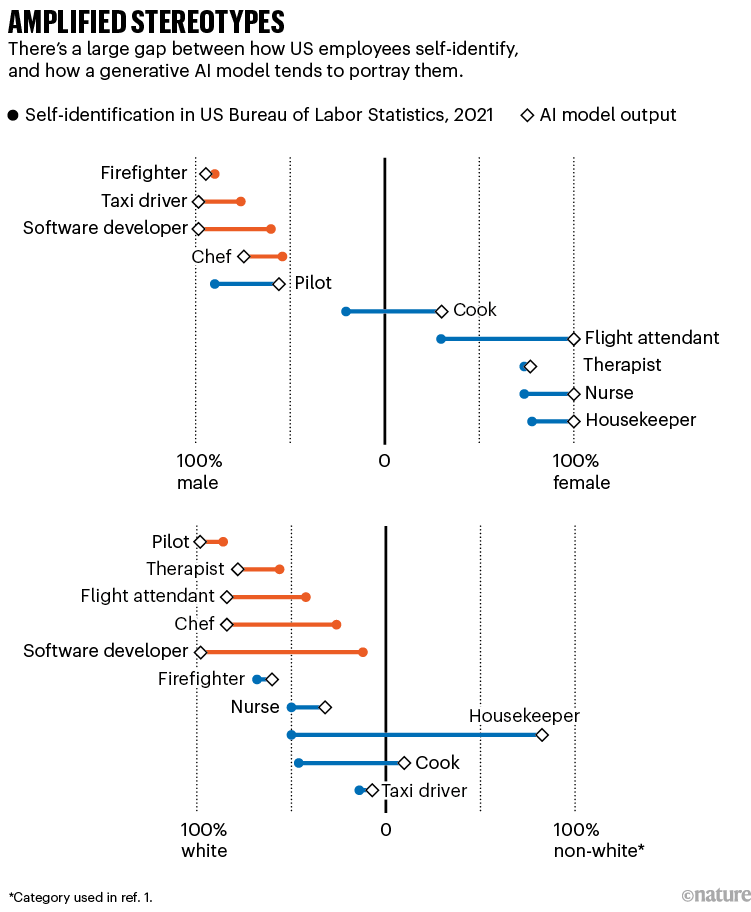
Source: Ref. 1
In 2017, eight Google researchers created transformers, the neural-network architecture that would become the basis of most AI tools, from ChatGPT to DALL·E. Transformers give AI systems the ‘attention span’ to parse long chunks of text and extract meaning from context. “It was pretty evident to us that transformers could do really magical things,” recalls computer scientist Jakob Uszkoreit who was one of the Google group. Although the work was creating a buzz in the AI community, Google was slow to adopt transformers. “Realistically, we could have had GPT-3 or even 3.5 probably in 2019, maybe 2020,” Uszkoreit says.
[ad_2]
Source Article Link