[ad_1]
When it comes to single-cell gene-expression data, biologists face an embarrassment of riches. There are thousands of data sets to choose from. Unfortunately, those data sets have not all been processed in the same way; they might use different names for similar or identical cells or tissues; and they are scattered across the Internet — or available only on request.
Smart software untangles gene regulation in cells
Using any one data set is relatively straightforward. But collecting, curating and integrating the data to draw conclusions across experiments, is — in the words of bioinformatician Timothy Triche Jr at the Van Andel Institute in Grand Rapids, Michigan — “a huge pain in the butt”.
In one 2023 study1, for instance, computational biologist Christina Theodoris at Gladstone Institutes in San Francisco, California, described a deep-learning model called Geneformer. Building on some 30 million single-cell transcriptomic data sets that Theodoris manually aggregated in 2021, Geneformer allows researchers to predict the impact of gene perturbations in cell types or genes it has never seen. But because the data were scattered across 18 public databases and multiple independent laboratories, she says, “it took me two months to collect all that data and process it”.
A vast resource
Today, the same effort would take only minutes, she says, thanks to a new resource from the Chan Zuckerberg Initiative (CZI) in Redwood City, California. Chan Zuckerberg CELL by GENE Discover (CZ CELLxGENE) is a collection of free and open-source tools for finding, querying, analysing, downloading and publishing single-cell data. As of April, it includes some 85 million single cells and 1,317 data sets covering 844 cell types, curated and uniformly processed by a team of 25 or so engineers, data curators and other staff, according to Patricia Brennan, vice-president of science technology at CZI. Most of the data represent single-cell RNA sequencing information from healthy human tissues, but non-human and cell-line data, as well as molecular-profiling data obtained using spatial transcriptomic methods, are also available. All of these data are stored in a common format, using a standard set of cell types and metadata.
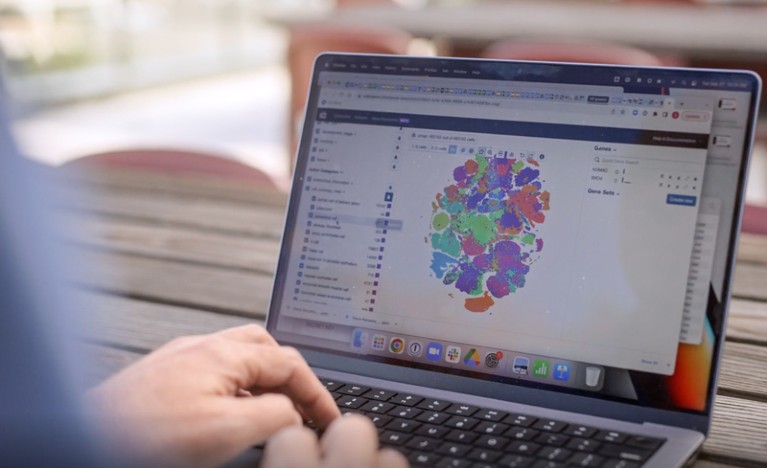
The CZ CELLxGENE tool helps researchers to visualize gene-expression data.Credit: Chan Zuckerberg Initiative
Users can find and explore the non-spatial data through the CZ CELLxGENE data portal, or access it using the R or Python programming languages through an application-programming interface called Census. (Spatial data should be added later this year, a spokesperson for CZI says.) Meera Prasad, a graduate student at the California Institute of Technology in Pasadena, is using CZ CELLxGENE to characterize the microenvironment across 9 million healthy and cancerous mammary cells representing some 150 cell types. By integrating those data with her lab’s spatial data, Prasad hopes to better replicate the tumour microenvironment, and also to identify genes that are related to the structural changes associated with cancer.
How to make spatial maps of gene activity — down to the cellular level
CZ CELLxGENE enables two key applications, says Jonah Cool, a science programme officer at CZI. Most obviously, researchers can ask questions across a vast amount of data that they and others have collected. Triche, for instance, has plumbed some 12 million mouse cells to study the influence of sex chromosomes on the biology of immune cells. “That’s approximately 11-and-a-half million more cells than we would typically run in a single-cell experiment,” he says. Repeating those analyses in-house would be a waste of money, but leveraging data that others have processed can be tedious. By ‘harmonizing’ these data sets and putting them in one place, CZ CELLxGENE removes many of what Triche calls “schlep steps”. “People underestimate the degree to which the impact of this data is amplified by making it usable for anybody who wants to,” he says.
The other application is in artificial intelligence. Researchers can use CZ CELLxGENE to build and train computational models that can predict, for instance, the identity of a cell or the impact of specific perturbations.
Model modularity
Users can select any of five such models, including Geneformer, and refine or apply them to their own data. They can also download ‘embeddings’ — compressed numerical representations of transcriptional data — from any of them, allowing users to ‘project’ their data and CZ CELLxGENE data into a common space. That, says Cool, means researchers can ask questions such as what cells are similar to a researcher’s cells, or which conditions induce changes in those cells.
Computer scientist Jure Leskovec at Stanford University in California, used his Universal Cell Embeddings model2, which he trained on CZ CELLxGENE data, to identify rare mouse kidney cells known as Norn cells. By then applying this ‘classifier’ to a larger data set of 36 million cells, he found that Norn cells were also present in the heart, lung and gonads. “This generalizability is the key capability of these models,” he says.
NatureTech hub
CZ CELLxGENE is not the only resource that aggregates and simplifies single-cell data analysis. The Human Cell Atlas, for instance, has its own data portal. And both the University of California, Santa Cruz, and the Broad Institute of MIT and Harvard in Cambridge, Massachusetts, among others, host tools for analysing select single-cell data sets online.
In March, Lior Pachter, a computational biologist at the California Institute of Technology, and his team described their Commons Cell Atlas infrastructure3,4 that stores and uniformly processes raw sequence data across data sets. (By contrast, CZ CELLxGENE retains data as ‘gene-count matrices’, although links to the original sequence data are also maintained, a spokesperson says.) These sequence data can be reanalysed as gene annotations change, Pachter notes, and his team exploited that to study gene-splice isoforms in human testis. “It’s really powerful and useful to be able to go back and rebuild the atlas again and again and again,” he says.
In September 2023, CZI announced that it would build a computing cluster of 1,000 graphical processing units (GPUs), which can rapidly accelerate or scale up model development.
This is helpful to researchers because most labs doing single-cell research, Cool explains, have access to maybe a handful of GPUs, therefore limiting the complexity of the models that they can build and lengthening experiments. Using the new cluster, Cool says, researchers can begin to build more sophisticated — and accurate — models. The cluster is expected to be “up and running by June”, a spokesperson says.
[ad_2]
Source Article Link