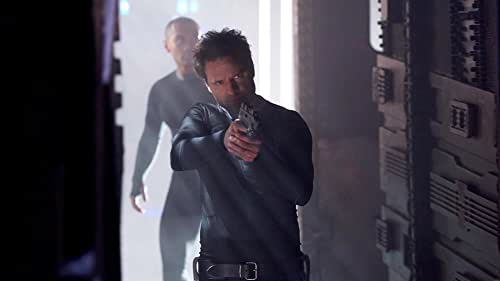
© Madrona Venture Group’s Smart Apps Summit powered by GeekWire Life Science Speakers . From left: Lucas Nivon, CEO of Cyrus Biotechnology, Jonathan Carlson, a researcher at Microsoft, Madison Masaelli, CEO of DipCell, and moderator Chris Picardo, Madrona’s business partner. (Photo by GeekWire/Charlotte Schubert)
Life scientists have a data problem: data is fragmented, ambiguous, and incomplete. And that makes it difficult to take full advantage of AI technology.
At the Smart Applications Summit hosted by Madrona Venture Group in Seattle last week, a group of researchers discussed the challenges of implementing artificial intelligence tools in the life sciences.
Artificial intelligence technology is changing the way companies do everything from selling products to routing packages. New AI “basic” models such as GPT-3 and DALL-E, which can generate new expressions or images, have been developed using excellent training tools from the web.
But in the life sciences, “data standardization is very difficult,” said speaker Madison Masaelli, CEO of DeepCell, a startup that visually analyzes and classifies individual cells.
Cell biology data is compromised by differences in sample collection, storage and processing, Massali said, making comparisons between data sets difficult. “From sampling to imaging, there are dozens of steps that lead to variability in the data,” he said.
Not all life science data is messy. Protein structures, for example, are represented in a standardized way in standard databases. This enabled artificial intelligence tools from DeepMind’s AlphaFold and University of Washington’s RosettaFold, which recently solved the long-standing problem of predicting protein folding. UW recently launched ProteinMPPN, an AI-powered protein design tool.
But even for proteins, there’s a lot of information behind the walls. Lucas Nivon, CEO of Seattle-based protein design startup Cyrus Biotechnology, said Cyrus has reached out to major pharmaceutical companies to share databases of antibody structures underlying many therapies. Hundreds of thousands of such structures are silent in various companies.
Nivon said all companies are interested in gathering information and discussing the process of sharing property objects. “Also, nobody wants to be the first big investor,” Nivon said.
Cyrus joined Amazon Web Services and other partners this summer to form OpenFold, an open-source protein design nonprofit, and is now talking to potential partners about sharing information about the structure of these antibodies.
“It has dark matter on its side. It really does,” Nivon said. And everyone admits it.
© Powered by Geekwire Protein rings are smoothed by artificial intelligence-based software from the University of Washington Institute for Protein Design. (IPD photo)
The reliability and bias issues that affect AI modeling in technical applications also affect the life sciences, but in different ways, the speakers said.
When the AI produces a paragraph that makes no sense, users can see it right away. Jonathan Carlson, who leads life science research and incubation at Microsoft Health Futures, part of the tech giant’s research arm, said it’s difficult to judge whether this indicates a misdiagnosis or an incorrect protein structure.
“Many of the problems we see in the life sciences are not unique, but very acute,” Carlson added.
Testing an AI product and then feeding the data back into the model sounds like it at first, but in the life sciences this process can be time-consuming. Cyrus is testing several proteins developed by his collaborators to create new transgenic mice, a process that could take more than a year. But Nivon’s team used high-throughput in vitro and cell filtration systems.
Nivon says efforts to optimize the filtering system will allow for faster improvements in artificial intelligence models. Capsida Biotherapeutics, which repeatedly designs and tests gene therapy designs using animal models, takes tissue to assess which actually reaches the right place in the body, he said.
Researchers want to better link biological data to clinical outcomes, but many obstacles remain, including privacy requirements, Massali said. “There is no single Google proxy that covers all the biological or health information in the world,” he said.
Carlson envisions a future in which more life science data is anonymized and moved into standard, interoperable formats. Finally, data from clinical trials and animal experiments can be effectively networked to develop new hypotheses and refine key research questions.
Carlson says there’s a big question: “How do we enable collaboration while respecting not only intellectual property, but also privacy? What’s the point of being able to build huge base models when we can’t even open the data?